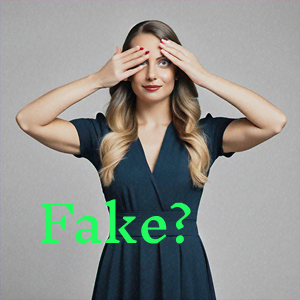
How AI Porn is Made: A technical overview
The creation of AI-generated adult content is a complex(well, everything is al ready here), multi-step process that involves deep learning, careful dataset management, and ethical considerations. In this article, I explore the main steps on how AI porn is technically produced. I’ll focus on the technical implications.
Dataset collection
Finally, that gazillion terabyte of porn comes in handy. Data collection! Every AI model start with data collection. In this case adult material. AI doesn’t know what it has, so you have to tell it. What I mean by that is; it doesn’t know that a movie consists of parts, the intro (some rando at the door with a pizza), some 69, some deep troughting, and the money shot. You have to tell it what is what. That is called labeling. Also annotate what styles (cartoon, realistic, etc.) the videos are. In this way the AI can learn what is what. And later, recreate it.
Model training
Training an AI model to generate adult content involves fine-tuning an existing generative model with a curated dataset. Popular models used for this purpose include: • Stable Diffusion • StyleGAN2/3 • DreamBooth (for personalizing models with specific styles or characters)
The training process works as follows:
- Data Preparation: Images are resized, normalized, and labeled to fit the model’s input requirements.
- Fine-Tuning: Instead of training a model from scratch (which requires huge resources), creators fine-tune a pre-trained model using a smaller, focused dataset. This adapts the model to generate content with specific styles, body types, or themes.
- Hyperparameter Tuning: Learning rates, batch sizes, and training steps are adjusted to optimize the model’s output quality without overfitting.
- Regular Evaluation: During training, developers regularly test the model by generating sample outputs to check for realism, style consistency, and unwanted biases.
⚠️ Note: Ethical guidelines and legal regulations must be strictly followed when training models on sensitive content.
Fine-tuning ensures the AI can produce highly detailed and realistic images aligned with the dataset it learned from
Prompt engineering
Prompt engineering is the art of writing effective, descriptive prompts to guide an AI model’s image generation.
Good prompt engineering is crucial because it tells the AI what to create in fine detail.
Key Techniques for Prompt Engineering:
Detailed Descriptions:
Add attributes like age range, body type, lighting, clothing (or lack thereof), environment, and artistic style.
Example:
A hyper-realistic photo of a beautiful adult woman, natural lighting, detailed skin texture, bedroom background, shallow depth of field, 8K resolution.
Negative Prompts:
You can also specify what you don’t want. This reduces errors like distorted faces or broken limbs. Example negative prompt:
blurry, low quality, bad anatomy, disfigured, extra limbs
Prompt Chaining:
Combine multiple smaller prompts to create layered complexity in the image.
Weighting Keywords:
Some engines allow you to weight certain words to prioritize them (e.g., using parentheses or brackets).
Prompt engineering becomes a critical creative skill, especially when you want consistent, safe, and high-quality AI-generated images.
Wrapping Up
So there you have it — the technical process behind AI-generated adult content in a nutshell. From collecting and labeling mountains of data, to training models and crafting the perfect prompts, it’s a world that’s as complex as it is fascinating.
If you’re diving into this at home, remember: • Stay ethical • Stay legal • Respect people’s rights and privacy
AI gives you crazy creative powers — but with great power comes great responsibility (and yes, that applies even if you’re training an AI pizza guy). 🍕